Too hard. Too soft. Just right. It’s a tale as old as time, always searching to find the “just right”. It is usually about a girl with golden locks looking for which bed is “just right” in the home of three bears. But in our VacCAP story, Dr. Juan Zalapa’s team steps into the starring role, looking for ways to measure texture traits in cranberry that are “just right”.
“The main objective of this study was to identify what is the best way to evaluate texture in cranberry. Texture traits are the most important traits in cranberry for the industry and the research [community],” Hector Lopez-Moreno, a PhD student in Zalapa’s lab, said. “But we don't have a standard methodology to evaluate these kinds of attributes. We focus on these traits, based on different approaches.”
Zalapa and Lopez-Moreno have detailed their research in their latest paper, “A Survey of Key Methods, Traits, Parameters, and Conditions for Measuring Texture in Cranberry”.
“Currently, the industry is measuring texture based only on force—the amount of force you need to compress the fruit. But texture is more complex than that,” Lopez-Moreno said. “We use different approaches to evaluate these attributes based on puncture, shearing, and other different methodologies, in order to have a more complete picture of what is the difference between cultivars about these attributes.”
Firmness is one of the traits that the industry considers important for sweetened dried cranberry (SDC) production, and how they determine what fruit is more suitable to go through the SDC process. The less firm a fruit is, the less likely it will make it to the end as a quality final product.
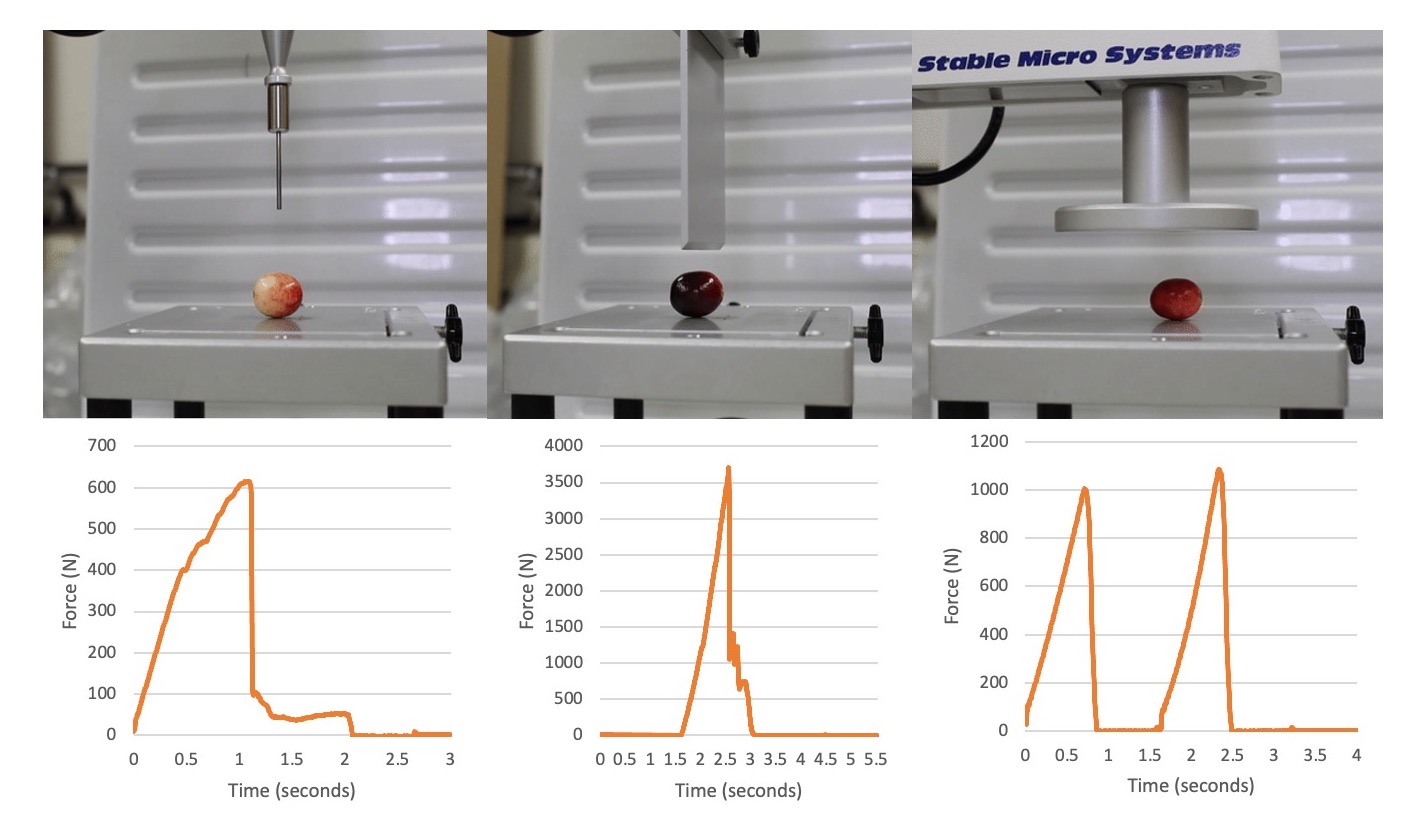
“When the fruit is not firm, it disintegrates during the processing,” Zalapa said. “There's a lot of mechanical agitation, there's a lot of soaking, heating, all kinds of different processes that go into making [SDCs], that by the time it gets to the other side of the line, there is a loss.”
According to anecdotal accounts there could be up to 50% loss of cranberries in the SDC production line. While there are several characteristics believed to impact this process—fruit size, internal structure, and color, etc.—Zalapa and his team focused on measuring firmness in this first paper.
“This is more of a survey of many different ways of doing the firmness measurements, and then playing with the parameters that we can change in the test.” Zalapa said.
The survey looked at several different key methods, traits, parameters, and conditions for measuring texture in cranberry.
- Methods: using different probes in the texture analyzer. Here they used single compression, double compression, puncture, shearing, and Kramer shear cell.
- Traits: traits are created out of the formulas and raw data gathered from the methodologies (there are 47 in this study).
- Parameters: have to do with the machine itself, different aspects of the tests. In this study, those were the speed at which the probe goes down, and strain—the percentage of the size of the fruit to which the probe has to go down.
- Conditions: aspects like temperature, fruit orientation and storage time that factor into results.
Some aspects of the survey were the first of their kind. Zalapa explained that nobody has ever conducted a double compression test, and that a single compression test is the only method that has been used in cranberry. A double compression test provides two different sets of data on a fruit’s texture allowing for more comprehensive trait analysis, while the industry has traditionally only been using one.
“Essentially, the industry has been using one trait, and now we have the ability to calculate dozens of traits that relate to this [first compression] peak, and this second [compression] peak,” Zalapa said.
According to Zalapa, the industry has traditionally used the raw data that comes from the single compression test. In this survey, Lopez-Moreno has been taking the data from all of their tests and putting it into models and formulas. This allowed for using specific parameters and conditions, which was also a new concept.
“Before this study, all the other authors that reported on textural firmness evaluation in cranberry, they used, I would say, kind of random parameters or conditions. But no one had investigated what is the best way to do it,” Lopez-Moreno said. “The values you can get from the textural machine can be widely different just because you test the fruits in different orientations, or if you use a different speed of the probe. Even if the values should be the same, it is misleading because you're using different parameters.”
Lopez-Moreno gave the example that if someone in the industry is using the speed of three millimeters per second, and another is using 10 millimeters per seconds, probably the firmness values will be quite different.
“He's going to report probably higher values compared to the second just because of the difference of the parameters. Not because the fruit is firmer itself,” Lopez-Moreno said. “That's why we have to develop and understand the methodology in order to evaluate these traits in the same conditions and to have resources that are comparable to each other.”
This survey is the first step in creating a universal standardization in measuring cranberry texture traits. Variability of equipment, measurements, and priorities are some of the things that led the Zalapa lab to take a closer look.
Everybody using different machines is an issue. According to Zalapa, the most used machine is automated, meaning there is no way to adjust any parameters. It was also developed for grapes and blueberries, much softer fruits that require less pressure from the machine. If the machines set up for softer fruits try to measure cranberries, they may not have enough pressure to accurately determine its firmness.
Zalapa knows they can’t make everyone use the same machine—despite that being the ideal scenario—but they at least want to make the industry aware that there are machines that are adjustable and those that are not, so, even if the machines are different they could be adjusted to use similar parameters.
It is important to use the same amount of compression force that the machine puts on a cranberry. Strain is the percentage of the size of the fruit to which it will be compressed. For example, if a fruit is one centimeter wide and it will be compressed at 10%, then the probe will go down 10 millimeters. The machine the industry typically uses has a strain of 2%. The Zalapa lab didn’t feel this was enough and set their minimum at 5%, testing upwards from there, determining the best strain is between 5% and 10%.
“Anything higher, the cranberry starts developing cracks. Anything below that is just not enough force to actually measure the firmness,” Zalapa said.
Also, it’s important to use a machine that can be outfitted with different probes. Probes used for puncture tests—another form of methodology—also vary greatly with at least 50 different types. Zalapa and his team narrowed that down to three different areas: compression, puncture, and shearing.
“It’s three different general methodologies, but each probe can be considered its own methodology. We wanted to choose more or less the probes to represent three broad areas,” Zalapa said.
The team also looked out how various conditions in the testing process could impact firmness data. Lopez-Moreno looked to define the optimal sample size for all the methodologies, finding 30 fruits to be a reasonable number.
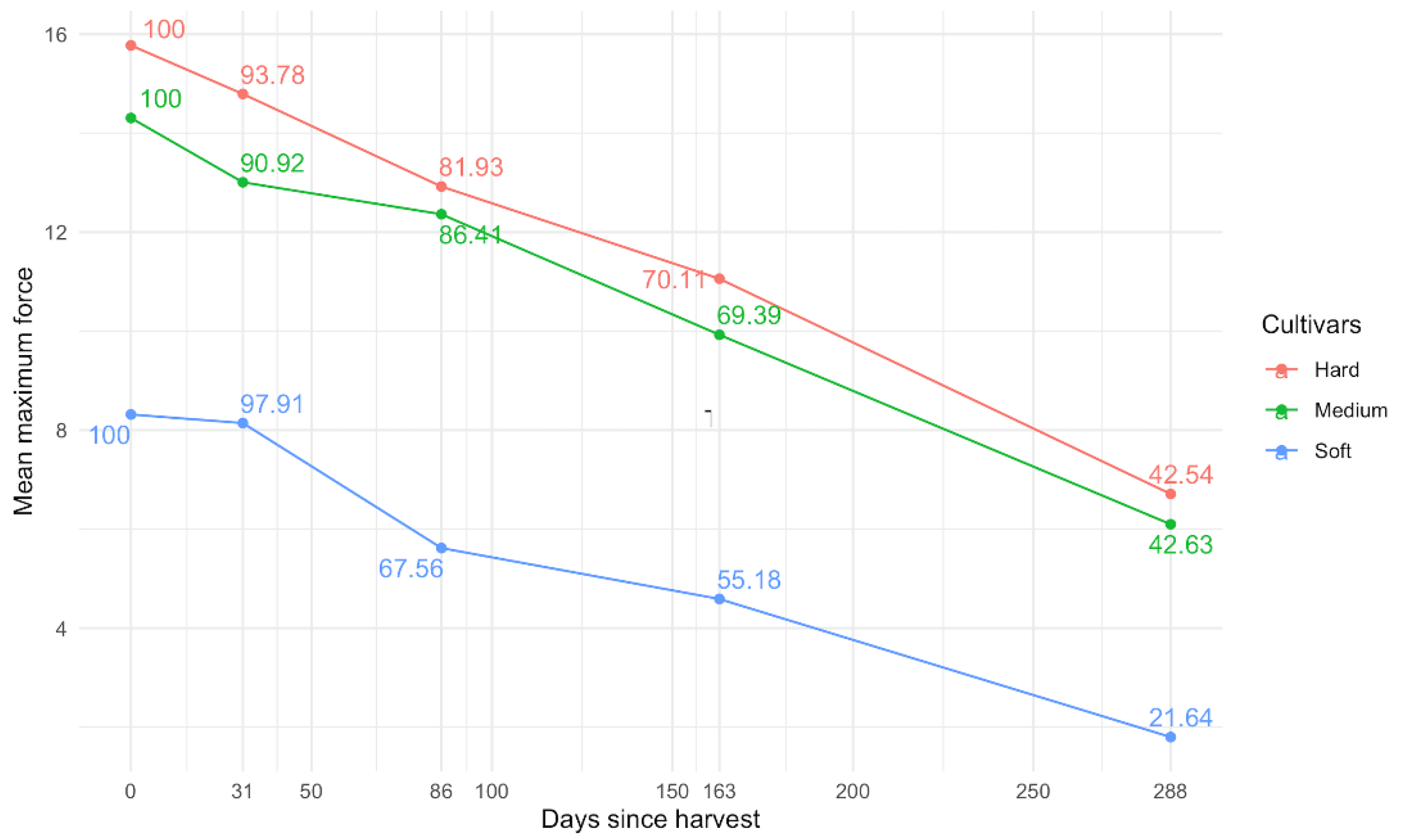
“In industry or other research [labs], they have been using 5 or 50 fruits of different sample sizes. But, if you use five fruits, you're unable to get an accurate measurement because there's a lot of variation,” Lopez-Moreno said. “But if you are using 50 fruits, you probably get more accurate [data], but that many fruits are not necessary. You're just wasting fruits and time. That's why it's important to find the ultimate sample size.”
The effect of storage on fruit firmness is important to consider, since firmness decreases when the fruit is left in storage for extended periods of time. The team evaluated the firmness of fruit when harvested, and then after 31, 86, 163 and 288 days of storage. They found that medium or hard cultivars had similar trends where firmness decreased slowly at first with less than 10% firmness lost in the first 30 days and about 60% firmness loss at the end of the study. In contrast, soft cultivars had initial firmness of half of the firmer cultivars, but lost less firmness initially (~2%) and then lost ~80% firmness by the end of the storage period.
“The fact that during the first month, the decrease in firmness is slow, on average, it decreases less than 10%, is important because we are trying to measure a lot of fruit, and thus time is a crucial part of the process,” Lopez-Moreno said. “And the fact that the decrease is not too bad in the beginning is an indication that we have at least one month to get accurate results.”
Lopez-Moreno mentioned that understanding the effect of fruit size on the performance of different parameters is key, since bigger fruits are usually firmer. It is something the team feels needs to be standardized between breeding programs and the industry, who have different sorting and weight requirements for berries.
“We need to decide as an industry, what is the ideal size for SDC—what needs to be rejected and what needs to be the minimum. And then that way, when we measure firmness, we can just measure firmness on those berries,” Zalapa said. “When cranberries in the industry get sorted, they get sorted by size. But in breeding, we're always concerned about weight. I think these are things that people are not thinking [about]. These kinds of things are important.”
According to Zalapa, the industry transitioning to using the same machine or at least having the ability to input the same parameters would still be the most beneficial outcome. He believes the machines that people are using in the industry are “really not to the best standard that they need to be in order to do this kind of measurement.”
“Part of our impetus for this research is to show the industry that they need to move on to something better, and that this type of machine where you can adjust parameters, use different probes, and everything [is optimal].” Zalapa said. “We're hoping that they take this and they say ‘Wow, there's a lot out there instead of just measuring a 2% compression and this machine that we're using can’t adjust anything or use different probes and you can't get any traits’.”
Initially, the Zalapa lab pioneered the work with this type of machine in cranberry. Zalapa says the VacCAP team then decided to standardize with the same machine for cranberry and blueberry, with slight variations between the two.
“Even though [the blueberry industry] could use the older, less powerful machine, they can't calculate anything. Because they don't get a graph, all they get is a number of what the compression force is,” Zalapa said. “With the graph, we can understand the time that it took for that pressure to actually build, we can understand the slope, we can understand a lot of things that you can't [with the old machine]. Firmness is a lot more complicated than people think. And that's what we're trying to show here.”
Zalapa says that at least two processors in Wisconsin have bought similar adjustable machines as his team has been working on. They worked with the processors to transfer the technique of how they set up the machines, the parameters they’re using, and they're doing their own test.
“And now we are getting questions from other growers and other bigger operations to actually get access so they can do the same things that we're doing. The different cranberry breeders have also started to work on [similar machines]. And we can start standardizing this work across the industry and breeding groups.” Zalapa said.
There are more papers in the pipeline to delve further on impacts of characteristics such as fruit size, color, and chemical makeup. They also plan to specifically separate out the cultivars using the methodologies, which they did not intentionally do in this first paper, but there are some early indications of which trend firmer than others.
“Now that we learned how to adjust the machine, what [options are] out there, and what the conditions are, we're going to choose the best conditions, parameters, traits, and then we're going to separate cultivars,” Zalapa said. “Eventually, we're going to use that for genetic mapping to understand what the genes are that control these traits.”
Feature Article by Josie Russo, Hector Lopez-Moreno, Juan Zalapa, Amaya Atucha, and Lisa Wasko DeVetter